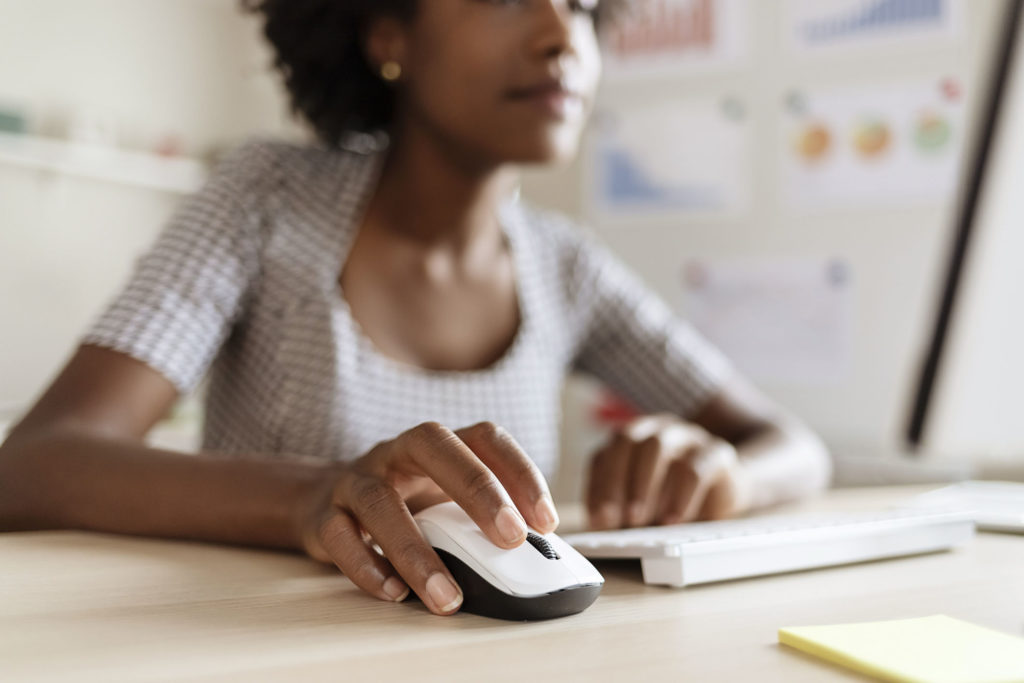
In our ever-increasing digital and automatized world, certain buzzwords are becoming more centre stage in the public sector. One of them is “artificial intelligence”. While the concept, and development, of artificial intelligence is not new (artificial intelligence was first recognised as a formal discipline in the mid-1950s), it is a word that has been casually thrown around more in recent years in the public sector, and sometimes carelessly.
Traditional algorithms vs machine learning models
These days modern data scientists normally associate with artificial intelligence systems that are based on machine learning models. Machine learning models deploy methods that develop rules from input data to achieve a given goal.1 There is a difference to, what you may call, traditional algorithms. Traditional algorithms don’t need data to learn, they just churn out results based on the rules inherent to them.
Traditional algorithms have been used in the public sector for some time to make decisions. The latest example making the headline was the model determining A level exam results last summer. From an auditing perspective, as the basis of the algorithms are usually transparent, auditing them is something we as a public audit institution are used to.2
But artificial intelligence that is based on machine learning is different – it has only been (cautiously) employed in the public sector in recent years.
It is different because, firstly, for a machine learning model to learn it needs good, quality data – and often a lot of it. Our report on the challenges of using data across government has shown that that condition is not always given.
Secondly, it can be quite costly to develop and deploy them. Moreover, the benefits are not always guaranteed and immediately realisable. In a public sector context with tight budgets, the willingness to put money behind them may not always be there.
The reason for this is related to a third point. It is not always certain from the outside what the machine will learn and therefore what decision-making rules it will generate. This makes it hard to state the immediate benefits. Much of the progress in machine learning has been in models that learn decision-making rules that are difficult to understand or interrogate.
Lastly, many decisions affecting people’s lives that artificial intelligence models would support pertain to personal circumstances and involve personal data, such as health, benefit or tax data. Whilst the personal data protection landscape has strengthened in recent years, there are not always the organisational regulatory structures and relevant accountabilities in place of the use of personal data in machine learning models.3 Public sector organisations are therefore at risk of inadvertently falling foul of developing data protection standards and expectations.
How to audit public sector machine learning models
Given all these challenges, it may not be surprising that in our public audit work, we are not coming across a lot of examples of the use of machine learning models in decision-making. But there are examples4 and we foresee that they may be growing in the future.
We have therefore teamed up with other public audit organisations in Norway, the Netherlands, Finland and Germany, and produced a white paper and audit catalogue on how to audit machine learning models. You can find it here: Auditing machine learning algorithms (auditingalgorithms.net).
As the paper outlines in more detail, we identified the following key problem areas and risk factors:
- Developers of machine learning models often focus on optimising specific numeric performance metrics. This can lead them to neglect other requirements, most importantly around compliance, transparency and fairness.
- The developers of the machine learning models are almost always not the same people who own the model within the decision-making process. But the ‘product owners’ may not communicate their requirements to the developers – which can lead to machine learning models that increase costs and make routine tasks more, rather than less time-consuming.
- Often public sector organisations lack the resources and/or competence to develop machine learning applications internally and therefore rely on external commercial support. As a result they may take on a model without understanding how to maintain it and how to ensure it is compliant with relevant regulations.
We also highlighted the implications for auditors to meaningfully audit artificial intelligence applications:
- They need a good understanding of the high-level principles of machine learning models
- They need to understand common coding languages and model implementations, and be able to use appropriate software tools
- Due to the high demand on computing power, machine learning supporting IT infrastructure usually includes cloud-based solutions. Auditors therefore also need a basic understanding of cloud services to properly perform their audit work.
Our audit catalogue sets out a series of questions that we suggest auditors should use when auditing machine learning models. We believe it will also be of interest to the public sector bodies we audit that employ machine learning models. It will help them understand what to focus on when developing or running machine learning models. As a minimum, it gives fair warning what we as auditors will be looking for when we are coming to audit your models!
Footnotes
- In fact there are two main classes of machine learning models. Supervised machine learning models attempt to learn from known data to make predictions; unsupervised machine learning models try to find patterns within datasets in order to group or cluster them.
- See for example our Framework to review models – National Audit Office (NAO) Report to understand more about what we look out for when auditing traditional models and algorithms. We currently have some work in progress that aims to take stock of current practices and identify the systemic issues in government modelling which can lead to value for money risks
- In the UK the Information Commissioner Office has published guidance on the use of personal data in artificial intelligence: Guidance on AI and data protection | ICO
- For some UK example see: https://www.gov.uk/government/collections/a-guide-to-using-artificial-intelligence-in-the-public-sector